https://www.nature.com/articles/s41467-021-24070-3
This work started with the problem that it’s hard to look at a cleft in a protein and predict if that cleft can do chemistry. We know there are millions of physical and chemical aspects of an enzyme that make catalysis happen. But which features could we use to predict catalysis? This is important for understanding enzymes. It’s also important for DESIGNING enzymes. Why? Well, it’s especially hard to predict if a designed enzyme cleft has activity or not because there are no markers of enzyme activity from evolution (because the amino acids in the cleft didn’t evolve they were designed).
We wanted to know if we could learn what the special sauce is that makes an enzyme active site catalytic.
Ryan Feehan and Meghan Franklin developed a machine learning model to tackle this challenge. When making our classifier the key decision was finding the non-enzymatic sites that we should train the model on. We decided to train the model to distinguish between metal binding sites and metal catalytic sites. That way the sites would be extremely similar other than that some could do catalysis and others couldn’t.
Making the dataset for this was a trial, but Ryan persevered on this task—taking it from an undergraduate research project through to a graduate research project! Ultimately, Ryan was able to find thousands of unique active and inactive metal binding sites. This dataset may be useful to others and so we made it available on github.
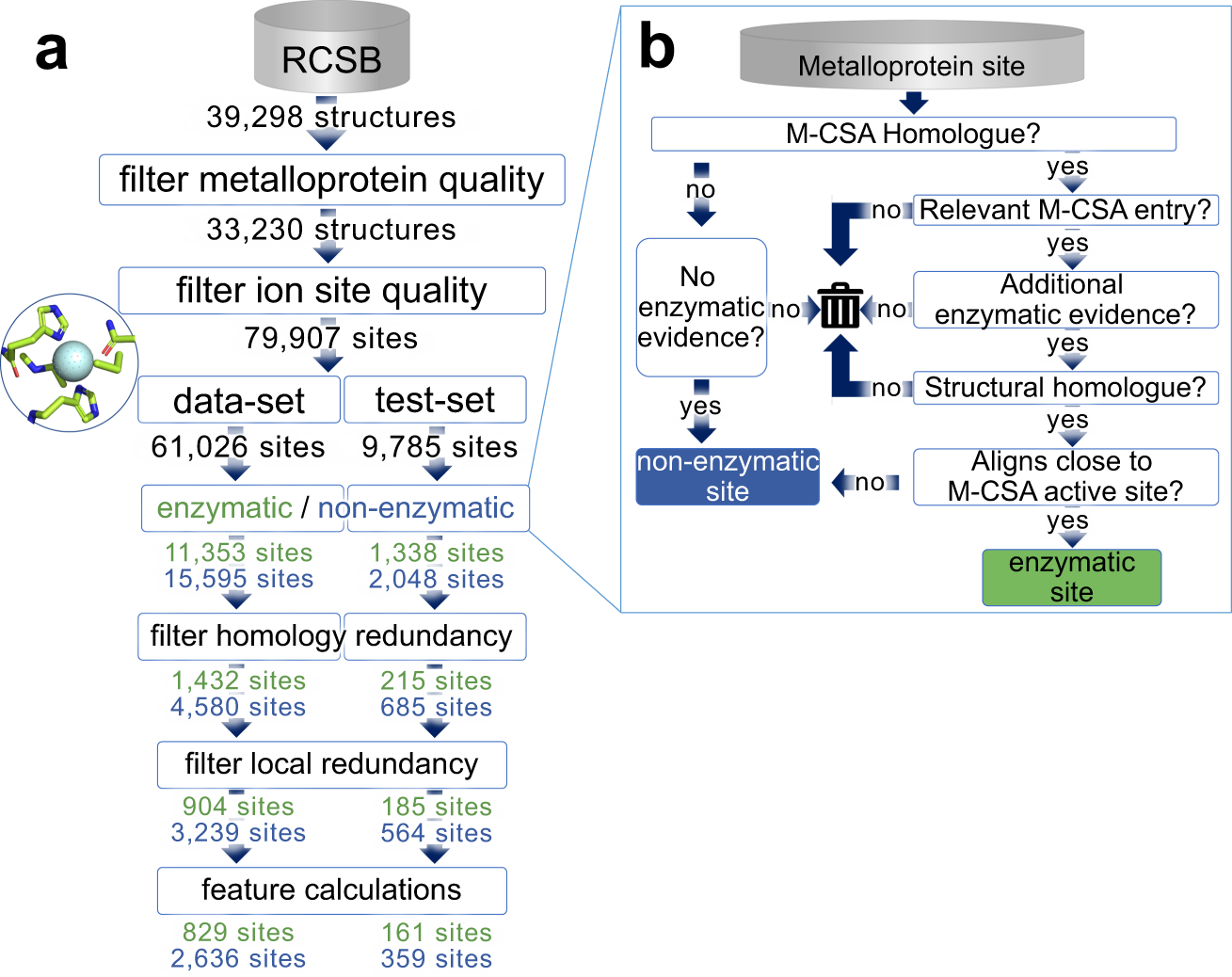
The sites were from all kinds of enzymes, giving us hope that this may lead to generalizable enzyme features.
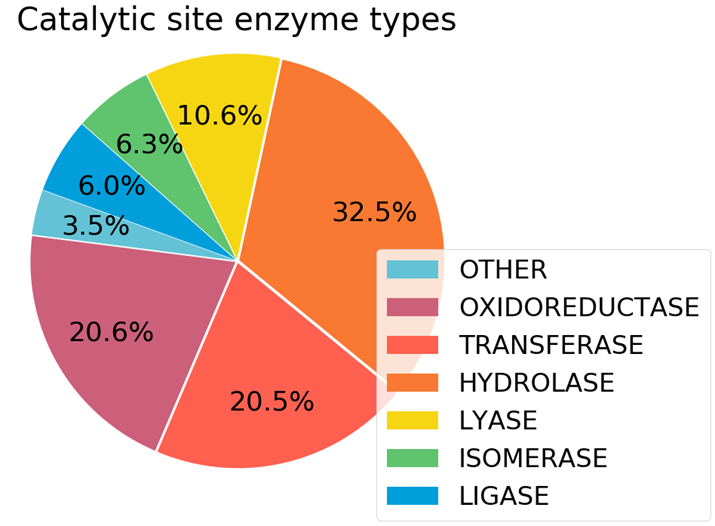
We tried all kinds of machine learning algorithms. Ryan named the top model Metal Activity Heuristic of Metalloprotein and Enzymatic Sites (MAHOMES). Incidentally, Ryan is a KC Chiefs fan. MAHOMES can distinguish between extremely similar metalloprotein sites and metalloenzyme sites with 92.2% precision and 90.1% recall using only physicochemical features!
We attribute this success to the training set we created which had two important characteristics:
1. The set was fairly balanced (more similar numbers of active and inactive examples)
2. The set used particular examples of active and inactive sites that were fairly similar to each other (all metal sites) so the model could pick out features that were truly important to catalysis
Overall, our physicochemical method compared well even in comparison to methods that used homology!
On a personal note, this is my first non membrane protein paper published in more than 20 years! I’m excited that the lab is going in new directions.